what are the advatanges to having more than one independent variable in an experimental design?
Chapter 8: Complex Inquiry Designs
Multiple Independent Variables
- Explain why researchers often include multiple independent variables in their studies.
- Define factorial pattern, and use a factorial blueprint table to represent and interpret uncomplicated factorial designs.
- Distinguish between principal effects and interactions, and recognize and give examples of each.
- Sketch and interpret bar graphs and line graphs showing the results of studies with unproblematic factorial designs.
Just as it is common for studies in psychology to include multiple dependent variables, it is besides common for them to include multiple independent variables. Schnall and her colleagues studied the effect of both disgust and private body consciousness in the same study. Researchers' inclusion of multiple contained variables in i experiment is further illustrated by the following actual titles from various professional journals:
- The Effects of Temporal Filibuster and Orientation on Haptic Object Recognition
- Opening Closed Minds: The Combined Effects of Intergroup Contact and Need for Closure on Prejudice
- Furnishings of Expectancies and Coping on Pain-Induced Intentions to Smoke
- The Effect of Age and Divided Attending on Spontaneous Recognition
- The Effects of Reduced Food Size and Package Size on the Consumption Behaviour of Restrained and Unrestrained Eaters
Just as including multiple dependent variables in the same experiment allows one to answer more research questions, so too does including multiple independent variables in the same experiment. For example, instead of conducting ane written report on the result of cloy on moral judgment and another on the effect of individual torso consciousness on moral judgment, Schnall and colleagues were able to conduct ane written report that addressed both questions. Only including multiple independent variables besides allows the researcher to answer questions about whether the outcome of one contained variable depends on the level of another. This is referred to as an interaction between the independent variables. Schnall and her colleagues, for example, observed an interaction between disgust and private trunk consciousness considering the effect of disgust depended on whether participants were loftier or low in individual trunk consciousness. As we will see, interactions are oftentimes among the nearly interesting results in psychological inquiry.
Factorial Designs
Overview
By far the most common approach to including multiple independent variables in an experiment is the factorial design. In a , each level of one independent variable (which tin can also be chosen afactor) is combined with each level of the others to produce all possible combinations. Each combination, then, becomes a condition in the experiment. Imagine, for example, an experiment on the consequence of prison cell phone use (yes vs. no) and time of day (day vs. night) on driving power. This is shown in the in Figure 8.1. The columns of the table represent cell phone use, and the rows stand for fourth dimension of day. The four cells of the table represent the four possible combinations or conditions: using a cell phone during the solar day, not using a cell phone during the day, using a cell telephone at night, and not using a cell phone at night. This particular blueprint is referred to as a 2 × 2 (read "two-by-ii") factorial pattern considering information technology combines two variables, each of which has 2 levels. If one of the independent variables had a third level (due east.1000., using a handheld cell phone, using a hands-free prison cell phone, and not using a cell telephone), then it would be a 3 × ii factorial design, and there would exist six distinct conditions. Notice that the number of possible conditions is the production of the numbers of levels. A 2 × 2 factorial design has iv weather, a 3 × ii factorial design has six conditions, a iv × five factorial design would have twenty weather condition, and and then on.
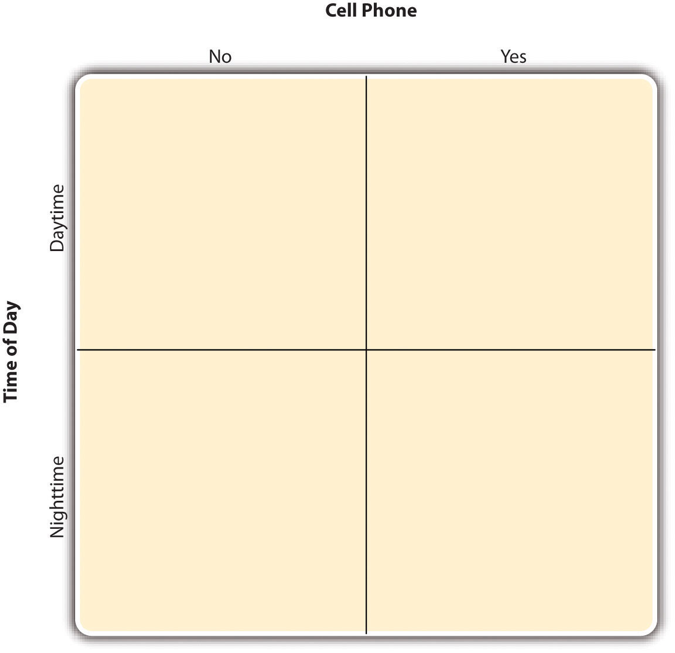
In principle, factorial designs can include any number of independent variables with any number of levels. For case, an experiment could include the type of psychotherapy (cerebral vs. behavioural), the length of the psychotherapy (2 weeks vs. 2 months), and the sex of the psychotherapist (female person vs. male). This would exist a two × 2 × ii factorial design and would have eight conditions. Figure 8.ii shows one way to represent this design. In practise, information technology is unusual for in that location to be more three contained variables with more than than 2 or three levels each. This is for at least two reasons: For 1, the number of atmospheric condition tin can quickly become unmanageable. For case, adding a fourth contained variable with iii levels (e.g., therapist feel: depression vs. medium vs. high) to the electric current instance would make information technology a two × 2 × 2 × 3 factorial design with 24 distinct weather. Second, the number of participants required to populate all of these conditions (while maintaining a reasonable power to discover a existent underlying consequence) tin render the pattern unfeasible (for more than information, see the discussion most the importance of adequate statistical power in Chapter 13). As a result, in the residual of this section nosotros will focus on designs with ii independent variables. The general principles discussed here extend in a straightforward way to more than complex factorial designs.
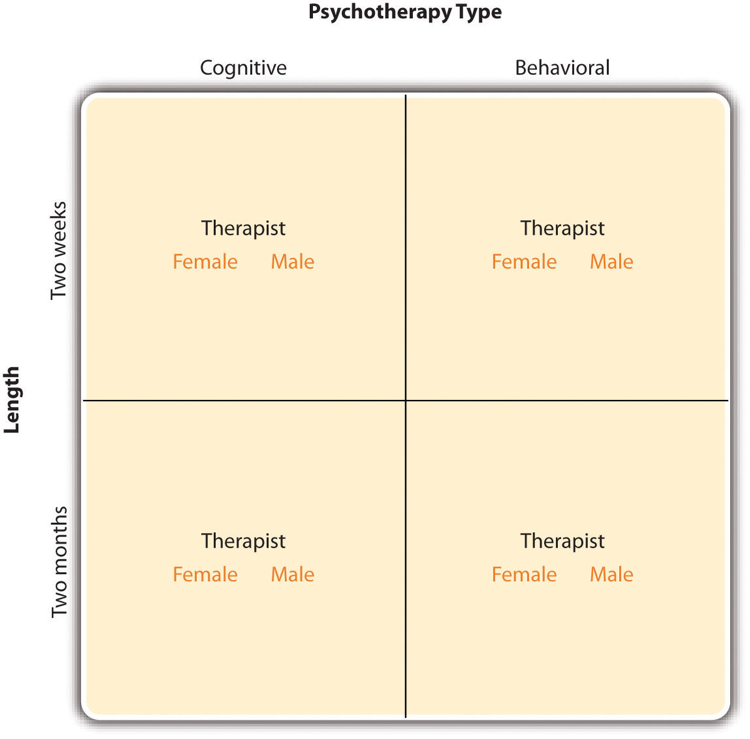
Assigning Participants to Conditions
Recollect that in a simple betwixt-subjects pattern, each participant is tested in merely one condition. In a elementary within-subjects pattern, each participant is tested in all weather. In a factorial experiment, the decision to take the between-subjects or within-subjects approach must be made separately for each contained variable. In a , all of the contained variables are manipulated between subjects. For example, all participants could be tested either while using a cell phoneor while not using a prison cell phone and either during the dayor during the night. This would mean that each participant was tested in one and but one status. In a within-subjects factorial design, all of the independent variables are manipulated within subjects. All participants could exist tested both while using a cell telephone and while not using a jail cell telephone and both during the dayand during the night. This would mean that each participant was tested in all conditions. The advantages and disadvantages of these two approaches are the same equally those discussed in Chapter 6. The between-subjects pattern is conceptually simpler, avoids carryover effects, and minimizes the time and attempt of each participant. The within-subjects design is more efficient for the researcher and controls extraneous participant variables.
Information technology is also possible to manipulate one contained variable between subjects and another within subjects. This is called a . For instance, a researcher might choose to treat cell phone use every bit a within-subjects factor by testing the same participants both while using a cell telephone and while not using a cell phone (while counterbalancing the order of these two conditions). Simply he or she might choose to care for time of twenty-four hour period as a between-subjects factor by testing each participant either during the mean solar day or during the night (perhaps because this only requires them to come in for testing once). Thus each participant in this mixed design would be tested in 2 of the four weather condition.
Regardless of whether the design is between subjects, within subjects, or mixed, the actual assignment of participants to conditions or orders of atmospheric condition is typically done randomly.
Nonmanipulated Contained Variables
In many factorial designs, i of the independent variables is a . The researcher measures it but does not manipulate information technology. The study by Schnall and colleagues is a skillful example. One independent variable was disgust, which the researchers manipulated by testing participants in a clean room or a messy room. The other was individual trunk consciousness, a participant variable which the researchers simply measured. Another example is a study by Halle Brown and colleagues in which participants were exposed to several words that they were later asked to call back (Brownish, Kosslyn, Delamater, Fama, & Barsky, 1999)[ane]. The manipulated independent variable was the blazon of word. Some were negative health-related words (e.k.,tumor, coronary), and others were not wellness related (e.g.,ballot, geometry). The nonmanipulated independent variable was whether participants were high or depression in hypochondriasis (excessive concern with ordinary bodily symptoms). The result of this written report was that the participants high in hypochondriasis were improve than those low in hypochondriasis at recalling the wellness-related words, merely they were no better at recalling the non-health-related words.
Such studies are extremely common, and in that location are several points worth making about them. Offset, nonmanipulated contained variables are usually participant variables (private trunk consciousness, hypochondriasis, cocky-esteem, and so on), and every bit such they are by definition betwixt-subjects factors. For example, people are either depression in hypochondriasis or loftier in hypochondriasis; they cannot be tested in both of these conditions. Second, such studies are generally considered to be experiments as long as at least ane independent variable is manipulated, regardless of how many nonmanipulated independent variables are included. Third, it is of import to remember that causal conclusions tin can only be drawn virtually the manipulated contained variable. For example, Schnall and her colleagues were justified in concluding that disgust affected the harshness of their participants' moral judgments considering they manipulated that variable and randomly assigned participants to the make clean or messy room. Just they would not have been justified in terminal that participants' private body consciousness afflicted the harshness of their participants' moral judgments because they did not dispense that variable. It could be, for instance, that having a strict moral code and a heightened awareness of one's body are both caused by some 3rd variable (e.yard., neuroticism). Thus it is important to exist aware of which variables in a study are manipulated and which are not.
Graphing the Results of Factorial Experiments
The results of factorial experiments with ii independent variables can be graphed by representing one contained variable on thex-axis and representing the other by using different kinds of bars or lines. (They-centrality is always reserved for the dependent variable.) Figure 8.3 shows results for two hypothetical factorial experiments. The top panel shows the results of a 2 × 2 design. Fourth dimension of day (day vs. dark) is represented by different locations on theten-axis, and jail cell phone use (no vs. yes) is represented by different-coloured bars. (It would also be possible to represent cell phone use on the10-centrality and time of day as unlike-coloured bars. The pick comes downward to which way seems to communicate the results nearly clearly.) The lesser console of Effigy 8.3 shows the results of a 4 × ii design in which one of the variables is quantitative. This variable, psychotherapy length, is represented forth thex-axis, and the other variable (psychotherapy type) is represented past differently formatted lines. This is a line graph rather than a bar graph because the variable on the 10-axis is quantitative with a small number of distinct levels. Line graphs are also appropriate when representing measurements fabricated over a fourth dimension interval (as well referred to as fourth dimension series data) on the x-axis.
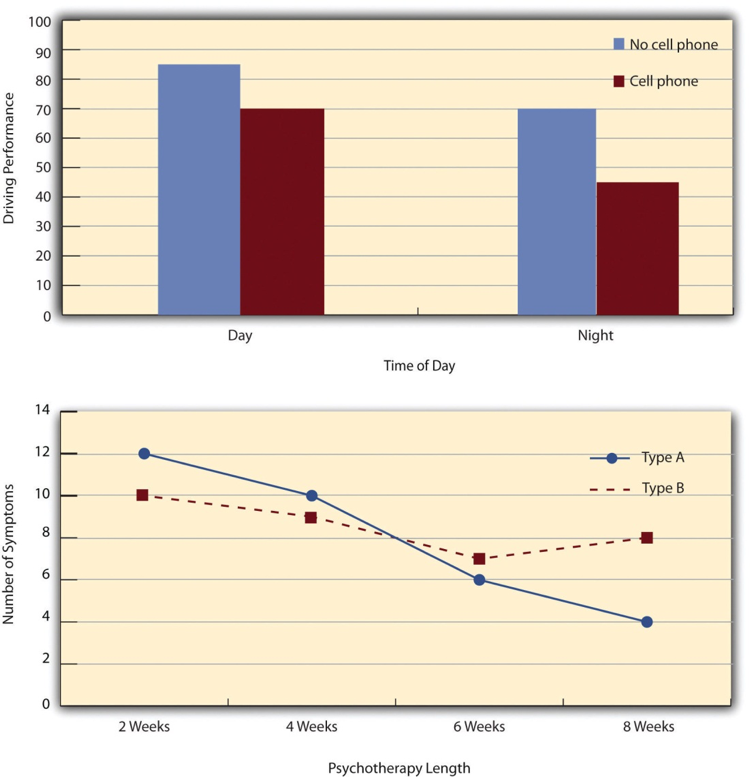
Main Effects and Interactions
In factorial designs, there are two kinds of results that are of involvement: main effects and interaction furnishings (which are also simply chosen "interactions"). A is the statistical relationship between i independent variable and a dependent variable—averaging beyond the levels of the other contained variable. Thus there is 1 main effect to consider for each independent variable in the study. The top panel of Figure viii.3 shows a chief effect of cell phone utilise considering driving performance was amend, on boilerplate, when participants were not using cell phones than when they were. The bluish bars are, on average, higher than the ruby-red bars. It also shows a main effect of time of day because driving operation was better during the day than during the night—both when participants were using cell phones and when they were not. Main effects are contained of each other in the sense that whether or not in that location is a chief effect of i independent variable says nothing about whether or non there is a primary effect of the other. The bottom panel of Effigy 8.3 , for example, shows a clear main issue of psychotherapy length. The longer the psychotherapy, the better it worked.
At that place is an effect (or only "interaction") when the effect of one independent variable depends on the level of another. Although this might seem complicated, you already have an intuitive agreement of interactions. It probably would not surprise you, for example, to hear that the consequence of receiving psychotherapy is stronger among people who are highly motivated to alter than among people who are non motivated to change. This is an interaction considering the effect of one independent variable (whether or not one receives psychotherapy) depends on the level of another (motivation to change). Schnall and her colleagues as well demonstrated an interaction considering the outcome of whether the room was clean or messy on participants' moral judgments depended on whether the participants were low or high in private body consciousness. If they were high in private trunk consciousness, then those in the messy room made harsher judgments. If they were low in individual body consciousness, so whether the room was clean or messy did not thing.
The effect of one independent variable tin can depend on the level of the other in several different means. This is shown in Figure 8.4 . In the pinnacle panel, independent variable "B" has an event at level 1 of contained variable "A" simply no effect at level two of independent variable "A." (This is much similar the study of Schnall and her colleagues where there was an outcome of disgust for those high in private torso consciousness simply not for those low in private torso consciousness.) In the middle panel, independent variable "B" has a stronger upshot at level 1 of independent variable "A" than at level 2. This is like the hypothetical driving example where there was a stronger result of using a cell phone at night than during the mean solar day. In the bottom console, independent variable "B" again has an upshot at both levels of contained variable "A," but the effects are in contrary directions. Figure 8.4 shows the strongest course of this kind of interaction, called a crossover interaction. One instance of a crossover interaction comes from a study by Kathy Gilliland on the effect of caffeine on the verbal examination scores of introverts and extraverts (Gilliland, 1980)[2]. Introverts perform improve than extraverts when they have not ingested whatever caffeine. Merely extraverts perform better than introverts when they have ingested 4 mg of caffeine per kilogram of body weight.
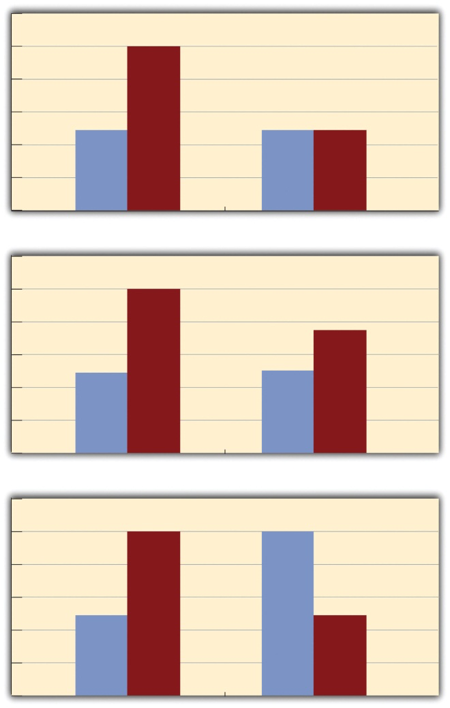
Effigy 8.5 shows examples of these aforementioned kinds of interactions when one of the independent variables is quantitative and the results are plotted in a line graph. Note that in a crossover interaction, the two lines literally "cross over" each other.
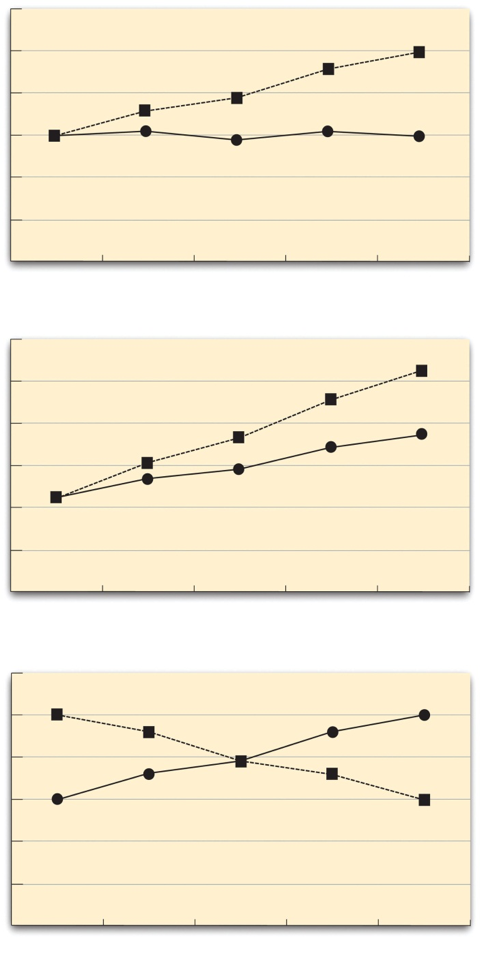
In many studies, the primary research question is about an interaction. The study by Brown and her colleagues was inspired by the idea that people with hypochondriasis are especially attentive to any negative health-related information. This led to the hypothesis that people high in hypochondriasis would think negative health-related words more accurately than people depression in hypochondriasis but recall non-health-related words about the same equally people depression in hypochondriasis. And of course this is exactly what happened in this study.
- Researchers often include multiple independent variables in their experiments. The most common approach is the factorial design, in which each level of one independent variable is combined with each level of the others to create all possible conditions.
- In a factorial pattern, the master event of an independent variable is its overall upshot averaged across all other contained variables. At that place is one main issue for each independent variable.
- There is an interaction between 2 independent variables when the upshot of one depends on the level of the other. Some of the most interesting inquiry questions and results in psychology are specifically about interactions.
- Exercise: Return to the five article titles presented at the beginning of this section. For each i, identify the independent variables and the dependent variable.
- Practice: Create a factorial pattern table for an experiment on the effects of room temperature and noise level on performance on the MCAT. Be sure to indicate whether each independent variable will exist manipulated between-subjects or inside-subjects and explain why.
- Practice: Sketch 8 different bar graphs to depict each of the following possible results in a 2 x two factorial experiment:
- No main effect of A; no chief outcome of B; no interaction
- Main effect of A; no chief result of B; no interaction
- No main issue of A; chief result of B; no interaction
- Main effect of A; chief effect of B; no interaction
- Principal event of A; main outcome of B; interaction
- Principal upshot of A; no main event of B; interaction
- No principal effect of A; main issue of B; interaction
- No primary effect of A; no primary result of B; interaction
Image Descriptions
Figure viii.v image clarification: Three panels, each showing a unlike line graph pattern. In the top console, i line remains constant while the other goes up. In the middle panel, both lines go up but at dissimilar rates. In the bottom console, one line goes down and the other goes up so they cross. [Return to Figure 8.5]
Source: https://opentextbc.ca/researchmethods/chapter/multiple-independent-variables/#:~:text=But%20including%20multiple%20independent%20variables,interaction%20between%20the%20independent%20variables.
0 Response to "what are the advatanges to having more than one independent variable in an experimental design?"
Postar um comentário